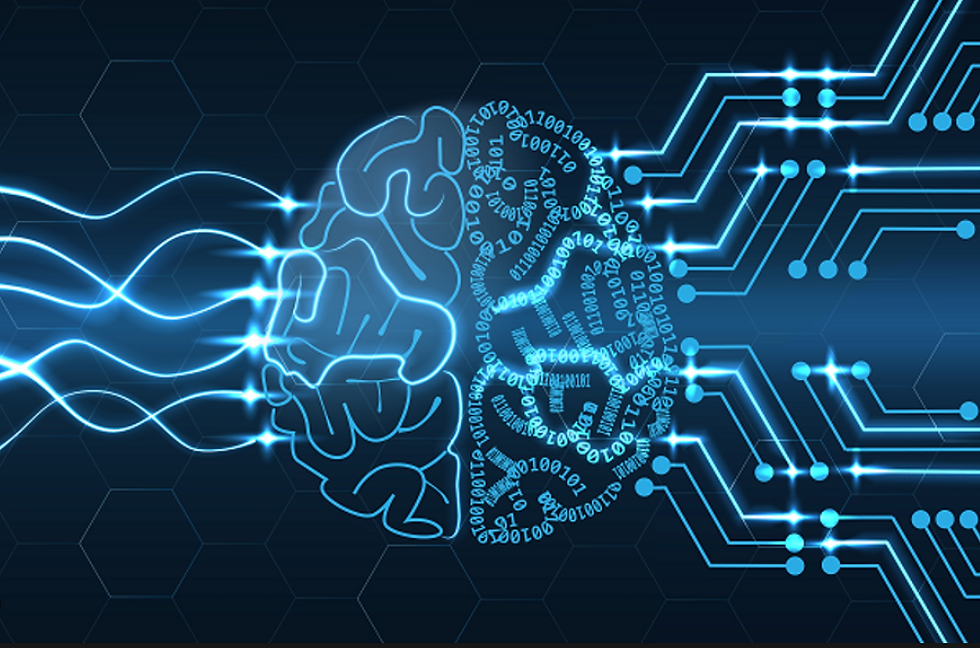
In the ever-evolving landscape of artificial intelligence (AI), few breakthroughs have captured our imagination like GPT-3. Developed by OpenAI, this massive language model stunned the world with its ability to generate remarkably coherent and contextually relevant text. Its 175 billion parameters catapulted it into the limelight, making it one of the most influential AI models to date. But here's the question that keeps many researchers, developers, and AI enthusiasts up at night: Can we replicate the success of GPT-3, and if so, what's the secret sauce?
GPT-3.5: The Gold Standard of AI Language Models
The success and popularity of GPT-3, GPT-3.5, and similar large language models developed by OpenAI can be attributed to several key factors that distinguish them from earlier models:
Scale and Size: OpenAI's models are exceptionally large and contain a vast number of parameters, which enables them to learn complex patterns and generate more coherent and contextually relevant text. GPT-3.5, for example, has 175 billion parameters, making it one of the largest models available.
Training Data: OpenAI trained its models on extensive and diverse datasets, encompassing a significant portion of the internet. This wide-ranging training data helps the models understand and generate content across various topics and domains.
Fine-Tuning: OpenAI fine-tunes its models on specific tasks and domains, which further refines their capabilities. Fine-tuning allows these models to excel in areas such as translation, summarization, chatbots, and more.
Research and Development: OpenAI invests heavily in research and development to advance the field of natural language processing. They continually refine and improve their models based on the latest research findings and techniques.
Accessibility: OpenAI has made its models accessible through APIs, making it easy for developers and organizations to integrate these powerful language models into their applications and services. This accessibility has driven their widespread adoption.
Ethical and Safety Considerations: OpenAI is proactive in addressing ethical and safety concerns associated with AI. They implement safety measures to prevent misuse, and they promote responsible AI use.
Innovative Training Techniques: OpenAI uses innovative training techniques, such as unsupervised learning and self-supervised learning, to improve the model's understanding of language and its ability to generate coherent text.
Collaboration and Feedback: OpenAI encourages collaboration with the developer community and gathers feedback to improve the models and tailor them to user needs.
Iterative Development: OpenAI has developed multiple iterations of GPT models, learning from previous versions' limitations and using that knowledge to create more capable and reliable models.
While foundation models existed before, OpenAI's success is due to their continuous research, large-scale infrastructure, dedication to safety, and their commitment to democratizing access to AI technology. The combination of these factors has made GPT-3.5 and similar models particularly powerful and influential in various industries and applications.
Can we repeat the similar level of success and popularity?
Other companies can certainly strive to achieve success and popularity with their own models through training and fine-tuning, but it's essential to recognize that replicating the exact level of success and popularity of models like GPT-3.5 is a multifaceted challenge. Here are some factors to consider:
Scale and Resources: Models like GPT-3.5 are incredibly large, with hundreds of billions of parameters. Replicating this scale requires substantial computational resources and infrastructure, which may not be readily available to all organizations.
Data Access: GPT-3.5 was trained on vast and diverse datasets, which significantly contributed to its performance. Access to high-quality, diverse training data and the legal and ethical considerations of data usage are significant factors.
Research and Expertise: Developing state-of-the-art language models requires a deep understanding of natural language processing (NLP) and the ability to conduct innovative research. OpenAI has assembled a team of leading experts in the field.
Fine-Tuning Expertise: Fine-tuning models for specific tasks and domains is a specialized skill. It involves access to relevant data and knowledge of transfer learning techniques.
Safety and Ethical Considerations: Responsible AI development and addressing ethical and safety concerns are paramount. OpenAI has made significant efforts to ensure the safe and ethical use of their models.
Iterative Development: OpenAI's success is also attributed to its iterative development process, which includes ongoing research and improvement based on feedback.
While achieving a similar level of success may be challenging, organizations can still work towards developing highly effective language models by:
Collaborating with the AI research community and leveraging collective knowledge.
Focusing on specific domains or use cases where their expertise and resources excel.
Prioritizing ethical AI development and responsible use.
Innovating in model architecture, training techniques, and fine-tuning strategies.
Gathering feedback from users and continually refining their models.
Success in AI and NLP can be measured in various ways, and organizations can make significant contributions and gain recognition in specific niches or applications even if they do not reach the same level of prominence as GPT-3.5.
Unlocking the Secrets
So, what can we do to unlock the secrets of replicating AI supermodels like GPT-3?
1. Scale Wisely: While emulating GPT-3's size might not be feasible for everyone, it's crucial to strike a balance between size and computational resources. Opt for a size that suits your objectives and resources.
2. Diverse Data: Curate diverse, high-quality training data to train your models. If access to extensive datasets is limited, focus on carefully curated data relevant to your objectives.
3. Research and Collaboration: Collaborate with the AI research community and share your findings. Leveraging collective knowledge can lead to innovative breakthroughs.
4. Responsible AI Development: Embrace responsible AI development practices. Address ethical and safety concerns to ensure the ethical use of your models.
5. User Feedback: Gather feedback from users and engage with the developer community. Use this feedback to continually improve your models.
6. Stay Agile: Stay agile in your approach. Adapt to the evolving field of AI and NLP research.
While replicating GPT-3's success is a daunting challenge, it's a path worth exploring. Learning from GPT-3's accomplishments and failures can pave the way for future supermodels, each unlocking new possibilities and reshaping the AI landscape. It's a journey that promises to redefine what we can achieve with AI and transform the way we interact with machines. So, let's embark on this quest to unlock the secrets and go "Beyond GPT-3."
OpenAI has charted a path for us all, and it's up to the AI community to follow in these giant footsteps, to not just replicate, but also to innovate and push the boundaries of what AI can achieve. The magic of GPT models lies in this journey, and the journey continues, promising to redefine the future of artificial intelligence.
Comments